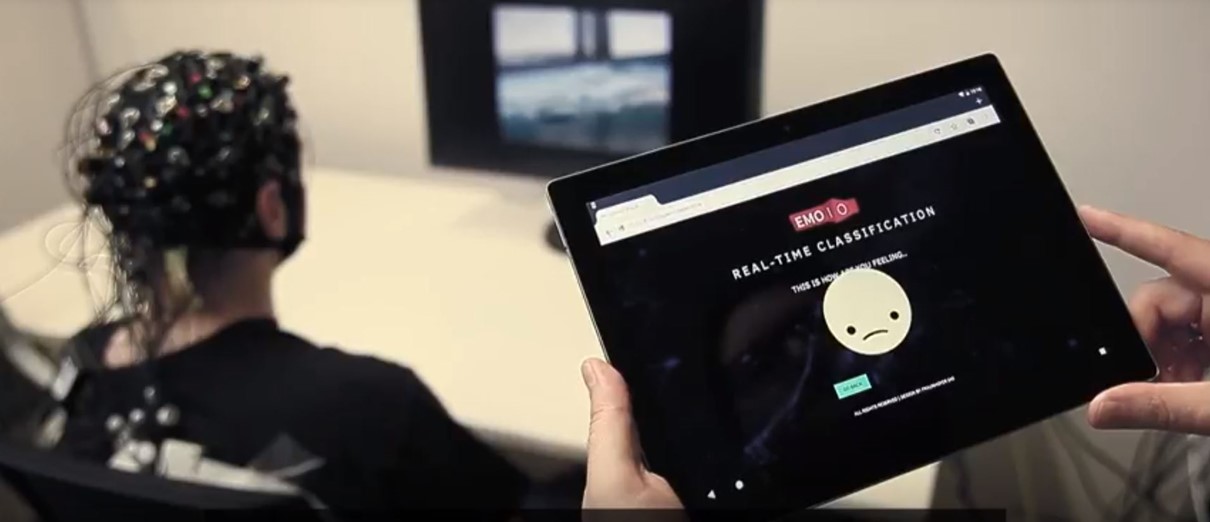
Today's assistance systems act more and more autonomously, usually without the possibility of direct feedback for users. The adaptation of assistance operations to the individual needs of the user through a brain-computer interface for affect recognition can reduce barriers for usage and promote acceptance.
Advances in sensor technology and artificial intelligence arise the possibility for technical systems to act ever more flexibly and autonomously. Examples range from a simple adjustment of screen brightness to the time of day and ambient lighting, to lane-keeping and distance assistants in vehicles, to cooperating industrial robots and service robots for usage at home. For the beneficial establishment of intelligent technology in contact with people, it is important that users' emotional states and preferences are also taken into account, appropriately. Only when a technology responds sensitively and promptly to its users’ intelligent systems will be perceived as cooperative and people-oriented and be accepted as a source of assistance or as a partner. For this purpose, it can be advantageous, for example, to continuously detect the affective reaction of users.
The EMOIO project had the objective of live detection of the subjectively perceived appropriateness of an adaptive system behavior in order to thereupon optimally adapt the assistance functions to the individual needs and preferences of the users. In the first phase of the project, positive and negative emotions were recorded using the neurophysiological methods electroencephalography (EEG) and functional near-infrared spectroscopy (fNIRS) during human-technology interaction and classified using machine learning methods in a so-called brain-computer interface. This information can be interpreted as an assessment of system behavior (agree/disagree and disagree/dislike). In the second phase of the project, mechanisms were developed to report the recognized affect reactions back to the adaptive system so that it can adjust its system-initiated assistance functions accordingly.
The project has conducted several studies on the identification of positive and negative affect using neurophysiological methods (EEG and fNIRS) in human-technology interaction. The studies included users of different ages. These served as a basis for creating an algorithm for live classification of affective user responses based on neurophysiological signals such as EEG and fNIRS. Furthermore, a mobile app was designed to display live classified affective responses to users. The functionality of the brain-computer interface has now been tested in realistic application scenarios. The application fields considered interactive robots, the smart home and driving in smart cars.
Emotion recognition via brain-computer interface